Home » Technology »
AI Has Come for Your Aircraft
Xwing President and CTO Maxime Gariel reveals how the aviator is unleashing AI technology to power up their aircraft
December 27, 2023 |
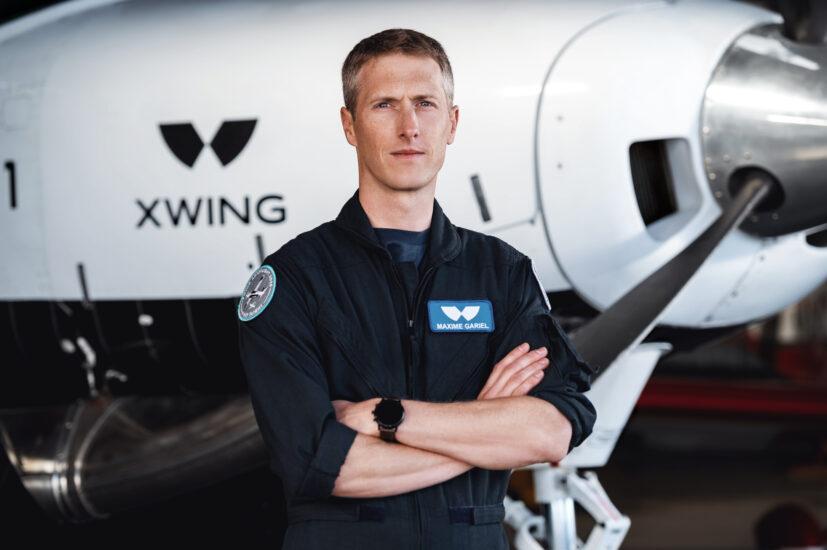
Xwing CTO Maxime Gariel is a pilot on a mission to create safe, fully autonomous airplanes.
You may have noticed that our lives — our digital lives and, by extension, practically every aspect of our offline lives — are about to undergo a radical transformation not seen since the advent of the internet.
That transformation is being powered by artificial intelligence (AI). AI is computers supercomputing and processing data faster and more comprehensively than ever before.
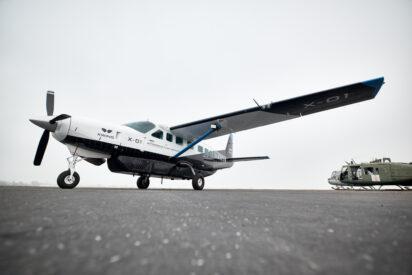
Xwing is the first Standard Category large unmanned aerial system (UAS) to receive official project designation from the Federal Aviation Administration (FAA).
One company taking advantage of AI to power its aircraft is California-based Xwing. Aerocar Journal (ACJ) recently checked in with Xwing president and CTO Maxime Gariel to learn how the aircraft developer has incorporated AI into its design and manufacturing processes.
ACJ: Maxime, is Xwing currently using AI in its development process?
Gariel: Often, when people think about AI, they think of ChatGPT or generative AI. This is not the aspect of AI we are focused on. We use a branch of AI known as machine learning — specifically deep learning — to enable the development of our autonomous flight software. We use this technology to solve problems in autonomy that cannot be resolved using existing methods.
Currently, we deploy AI-enabled software to provide redundancy and enhanced safety to solve problems that cannot be solved using traditional methods. One example is in vision-based landing. We use computer vision with machine learning-based systems to identify runways and ensure they are free of any obstacles before landing. Our AI-enabled software is bounded by physics and traditional methods.
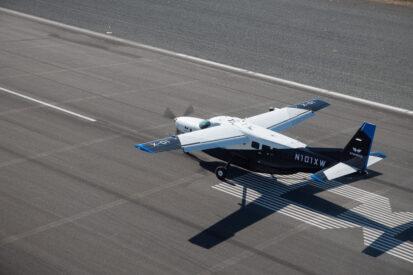
ACJ: What benefits do you hope to gain by using AI?
Gariel: AI solves the gap between highly automated and fully autonomous flight: Existing methods are well-suited for tasks like basic flight control, as has been shown by the long and successful history of autopilots and where using math and physics to solve the problem works well.
However, this only gets us about 95 percent of the way to full autonomy. To relocate the pilot, you have to solve that last 5 percent. AI-enabled methods are ideal for the aspects of pilot performance that are more difficult to condense into the mathematical equations. For instance, a lot of the pilot’s role is to look for obstacles and modify the aircraft’s trajectory to avoid them.
We are focused on deep learning, in which algorithms independently create models through repeated exposure to training data, rather than through explicit programming. For example, when you taxi, you have to detect obstacles — machine learning and deep neural networks work better than anything else we’ve seen.
AI adds redundancy and enhances safety. AI plays a safety-enhancing role by increasing the overall redundancy and performance of a system that also incorporates well-understood technologies by providing an alternate way to perform the same function. We are using it in a way that it’s always bounded by other methods so we can validate the output. For example, adding a vision-based landing system will ensure precision while protecting against GPS or ILS failures. It will bring higher levels of safety to small airports by allowing for auto-landings without the need for ILS infrastructure.
ACJ: Which company’s AI software are you using or planning to use?
Gariel: We have developed our own proprietary algorithms.
ACJ: Do you see any risks in using AI and, if so, how do you, or will you, mitigate them?
Gariel: We only use machine-learning based systems where existing methods will not suffice. We are not using ChatGPT-like generative AI where the outcomes can be unpredictable. We use deterministic methods, which means that we will test, and can accurately predict, how the software will react in different scenarios. We do not perform online learning. We have a rich data set of real-world flights, test flight data, and robust synthetic data to ensure our algorithms are trained to the highest safety levels.
For the certification of this technology, we are working closely with the [US] FAA on AI certification frameworks. Collectively, we are most interested in understanding the predictability and consistency of outcomes across the operational domain as we test our algorithms. We want to know under what parameters the technology will work as expected and when it will not so we can ensure the appropriate certification standards are developed.
Our recently announced collaboration with Daedalean AI shows our commitment to advancing each company’s AI algorithms and defining a common language and process for the certification of machine learning-based software. With a common understanding and certification standards, we can help facilitate the safe adoption of machine learning-based systems into our industry.
ACJ: Thank you, Maxime, for sharing this insight with our readers.
Gariel: Thank you