eVTOL Batteries: Advanced Machine Learning for AAM
Delft University Researchers Provide UAM Operators a Prognostics Tool for Battery Life
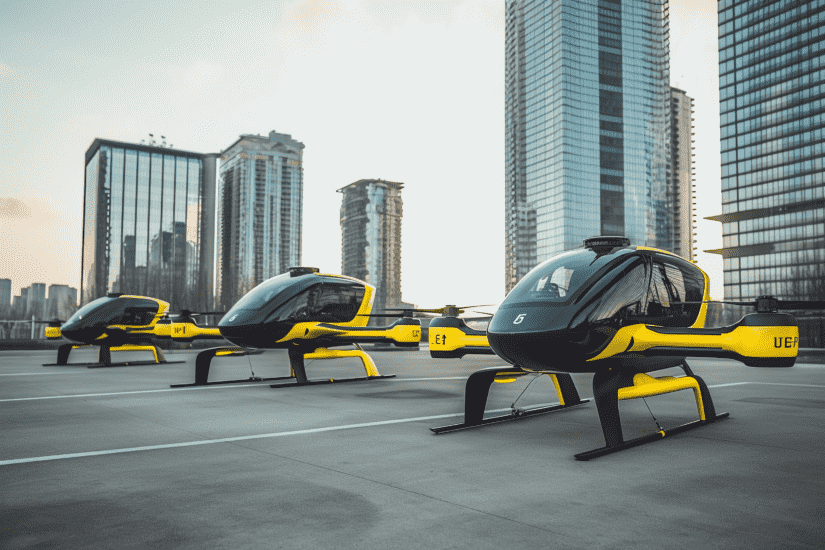
If you use your mobile phone frequently, when the remaining battery life reaches 20%, the battery indicator icon in the upper right of the screen turns red.
But if you’re operating your eVTOL the way many passenger-carrying operators expect to – lots of takeoffs and landings throughout the day, ferrying passengers from airports to downtown vertiports and back again – the batteries will undergo rigorous, varying load demands; discharge rates during takeoff require more energy than cruising, for example. Consequently, assessing and managing the health of a given aircraft’s batteries are critical to operations and ultimately the adoption of eVTOLs as they relate to urban environments.
A team of researchers at Delft University in the Netherlands proposed a data-driven machine learning protocol to discern the state-of-health and remaining useful lifetime of a given battery which considers the typical profile of an eVTOL’s flight patterns. Specifically, the scientists considered charge, discharge, and temperature.
They used five machine learning algorithms in their study: Support Vector Machine, Random Forest regression, Extreme Gradient Boosting, Gaussian process regression, and Multilayer perceptron. Among these, the most accurate method for state-of-health assessments proved to be the Random Forest regression while remaining useful life calculations were obtained using Extreme Gradient Boosting. The study also revealed that increasing cruise duration by up to 25%, Random Forest regression and Extreme Gradient Boosting were valuable as well.
Want to learn more about the emerging urban/advanced air mobility markets? Visit us at AeroCar Journal and subscribe today. It’s free (for a limited time!).
#aerocarjournal #aam #uam #evtols #ai #machinelearning