In Urban Air Mobility – Especially in Urban Air Mobility – Time Is Money
Real-Time On-the-Fly Motion Planning Can Save Pilots Pax, and UAM Operators Time and Money
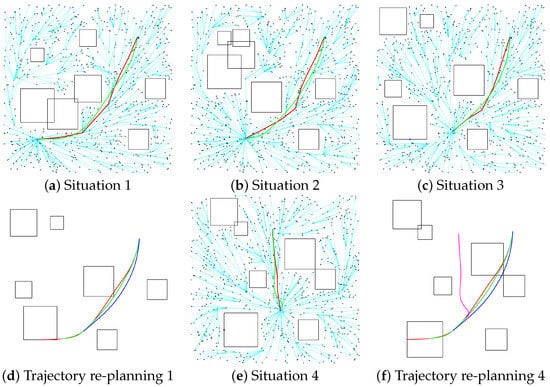
Your pax are onboard, you departed on-time. Your route was clear, weather was perfect and then – a geofence gets put up literally out of the clear blue sky. You want to minimize your snap trajectory and your trajectory but that’s easier said than done. Or it was, until a cohort of researchers from Cranfield University’s School of Aerospace, Transport and Manufacturing put on their thinking caps, took out their dry erase markers, and rolled out a whiteboard or two. OK, maybe it was an online collaboration, but Junlin Lou, Burak Yuksek, Gokhan Inalhan, and Antonios Tsourdos have a plan to keep you on schedule amid the myriad of uncertainties that can crop up midflight. They published their work in a special issue of Aerospace, Integrated Airborne Urban Mobility: A Multidisciplinary View.
Here’s the bottom line on how they’ve calculated not one, but two ways to improve your bottom line when your eVTOL operations are operational.
Take Your Pick: Option A or Option B
The Cranfield U scientists offered two ways to manage unexpected impediments pilots may encounter en route:
Option A uses a “decoupled method which aims for to design a policy network founded on a recurrent neural network for a reinforcement learning algorithm and then combining an online trajectory generation algorithm to obtain the minimal snap trajectory for the vehicle.”
Option B deploys “a coupled method using a generative adversarial imitation learning algorithm for training a recurrent-neural-network-based policy network and generating the time-optimized trajectory.”
Lou’s, Yuksek’s, Inalhan’s, and Tsourdos’s simulation reveals a short computation time when compared to other algorithms while still exploring the operating environment satisfactorily.